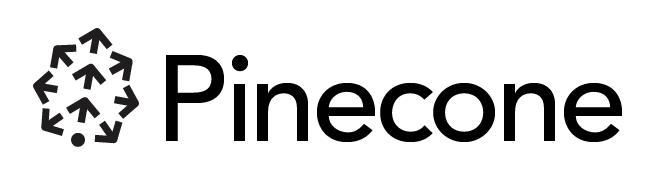
External reviews

External reviews are not included in the AWS star rating for the product.
Fine Product, Tough Debugging
The product overall is fine, but the GUI is quite disappointing when I try to debug. I have to write queries in the odd QL, which makes the entire process frustrating.
- Leave a Comment |
- Mark review as helpful
The fastest in production VectorDB yet
What do you like best about the product?
The speed. Hands down. QPS and the throughput is just the best in the industry. Easiest to get started with. Good support for parallel processing and batching.
What do you dislike about the product?
Nothing, just could release more complex document related retrieval systems.
What problems is the product solving and how is that benefiting you?
Semantic search is hands down a new way to search which is extremely efficient. Pinecone does a great job at not only providing the vector DBMS but giving the oppurtunity for scale.
Production-ready vector database to get you started quickly
What do you like best about the product?
- Good documentation and usage examples
- Easy-to-use Python SDK
- Production-ready with low latency at our scale (10-20M vectors)
- Good integration with the AI/LLM ecosystem
- Easy-to-use Python SDK
- Production-ready with low latency at our scale (10-20M vectors)
- Good integration with the AI/LLM ecosystem
What do you dislike about the product?
- did not find an easy way to export all vectors that we needed for data science/cleaning
- will get expensive when hosting 100s of millions of vectors
- will get expensive when hosting 100s of millions of vectors
What problems is the product solving and how is that benefiting you?
We use Pinecone as a vector database for retrieval augmented generation using LLMs.
A Reliable and Consistent Performance
What do you like best about the product?
Pinecone has been a game-changer for our company, especially in the realm of vector embeddings. What stands out the most is its robust performance and reliability. Over the six months of our usage, we have not encountered any downtime, which is crucial for our operations. The consistency in performance has been remarkable, ensuring that our data-driven processes run smoothly and efficiently. Its seamless integration have made it an indispensable tool in our tech stack.
What do you dislike about the product?
As of now, we haven't encountered any significant issues or drawbacks with Pinecone. It has met all our expectations and requirements efficiently. However, we are always on the lookout for new features and improvements that can further enhance our experience and capabilities with the platform.
What problems is the product solving and how is that benefiting you?
Pinecone has been instrumental in efficiently managing vector embeddings, a critical component in our applications like similarity search and recommendation systems. Its scalability and consistent performance, coupled with zero downtime, have significantly improved our operational efficiency and user experience. By simplifying infrastructure management and enabling rapid integration, Pinecone has allowed us to focus on core business functions, accelerating development cycles and enhancing overall service quality. This reliability and efficiency have been key to maintaining high service levels and staying competitive in our market.
User and Developer-friendly Vector Database that has helped our company scale
What do you like best about the product?
Pinecone has helped our company, fevr.io, scale our semantic chat functionality across three key regional markets. The responsiveness and ease of implementation has been a huge plus for our developers. The documentation has been very helpful as well, especially in terms of integrations with products like OpenAI and Langchain. Add to that, the customer support has been tremendously useful.
What do you dislike about the product?
While not necessarily a negative feedback, having even more research data on how different dimensions and pods affect various responses would be a helpful resource to have as a reference.
What problems is the product solving and how is that benefiting you?
Storing embeddings of documents is quite costly and difficult to manage. Pinecone solves this with solutions that are easy to implement with OpenAI's API. It allows for rapid prototyping of custom chat models.
Vector database that just works
What do you like best about the product?
We did a lot of research on vector databases at Refsee.com and tried many things: embedded db into the docker image served at AWS Lambda (believe me, that's not what you want), Milvus, Pinecone etc.
We always had problems and necessity of extra tuning before, both with self-hosted OSS dbs and managed ones, but Pinecone really did the trick! It just works!
We always had problems and necessity of extra tuning before, both with self-hosted OSS dbs and managed ones, but Pinecone really did the trick! It just works!
What do you dislike about the product?
As usual, if you choose managed solution you get a vendor lock. Probably can be costly if you scale and no option for on-prem installation
What problems is the product solving and how is that benefiting you?
We do vector search over our own datasets – basically a "google images" on our own data
Efficient and user-friendly, Ideal for vector database newcomers
What do you like best about the product?
I recently started using Pinecone and was impressed with how user-friendly it is, especially for someone new to vector databases. Its standout feature is its focus on doing one thing exceptionally well. The documentation is clear and easy to follow, making the setup process smooth. Both indexing and query times are impressively fast, which significantly enhances efficiency. I chose Pinecone over other options because it supports larger vector sizes, a key requirement for my needs. Highly recommend Pinecone for its simplicity, speed, and capabilities.
What do you dislike about the product?
There are a couple of areas where Pinecone could improve. First, the options for datacenter hosting are limited. For instance, if using AWS, it currently only supports the us-east-1 region, which can be restrictive. Second, the console lacks robust security measures for critical actions. Adding a Multi-Factor Authentication (MFA) verification for deleting indexes and projects would enhance security and prevent accidental data loss.
What problems is the product solving and how is that benefiting you?
Pinecone plays a crucial role in our workflow by efficiently storing vectors from OpenAI Embeddings. This capability allows us to effectively identify and link related content across various features of our platform. The result is a more cohesive and intuitive user experience, as we can seamlessly connect relevant information and offerings. This not only enhances our platform's functionality but also significantly improves user engagement and satisfaction.
Useful product for those who know what to do with it.
What do you like best about the product?
It is a fast and efficient vector database.
What do you dislike about the product?
The web-interface leaves many features to be desired.
It is quite a bit on the pricier side.
It is quite a bit on the pricier side.
What problems is the product solving and how is that benefiting you?
We use it to hold educational material
Highly recommend
Pinecone's vector database, with its exceptional performance and user-friendly interface, earns a solid five-star rating. It excels in managing large-scale vector data, offering both fast and accurate search capabilities. The integration process is straightforward, making it accessible for various applications, particularly in machine learning and AI-driven contexts.
Solid Hosted Vector DB
What do you like best about the product?
Ease of deployment! It takes just a few minutes to get an index set up and deployed.
What do you dislike about the product?
The web-based API console could be improved, for example for experiments with metric (cosine vs dotproduct vs euclidean).
What problems is the product solving and how is that benefiting you?
Storing embeddings for RAG.
showing 31 - 40